Archived Content
This page is archived and provided for historical reference. The content is no longer being updated, and some of the information may have changed over time and could be outdated or inaccurate.
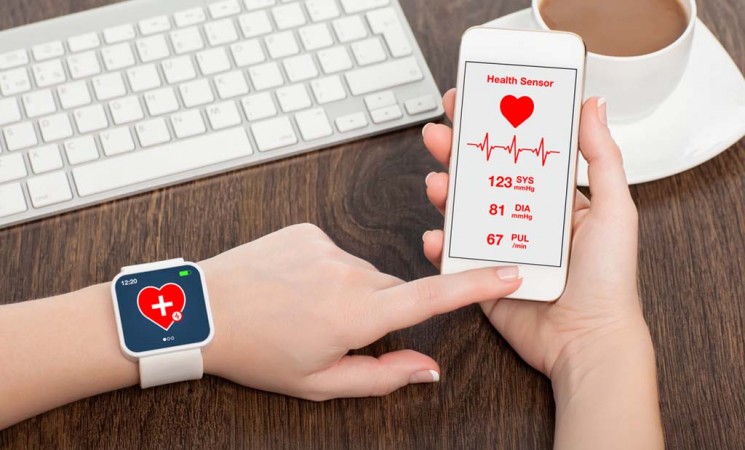
By Wendy Anson, Ph.D.
We live in a world where our wristwatch charts our morning path to work, measures our heart rate as we walk, jog, or run there, and then generates a report to tell us how well we “performed.”
With the increasing availability and use of mobile technology such as wearable computing sensors (e.g., activity monitors), a wealth of wearable “smart tool interactions” are now, quite literally, at the consumer’s fingertips. The health care industry has embraced the idea of wearable technology, and in the burgeoning field of eHealth, more and more applications are designed to detect and assess the physiological states of the person wearing or using them.
Many of these devices now feature an additional functionality: just-in-time adaptive interventions (JITAI) (Spruijt-Metz & Nilsen, 2014). As the name indicates, JITAI are dynamic and assess a suboptimal user state to then, on the fly, serve up appropriate intervention or support for the user.
Researchers have built these adaptive suites of interventions for a wide spectrum of behavioral health issues including alcohol use (Gustafson et al, 2014), smoking cessation (Riley, Obermayer, and Jean-Mary, 2008), and mental illness (Ben Zeev, Brenner et al. 2014). JITAI employ sensors designed to ferret out when a person is most vulnerable to unhealthy influences in their environment, and, perhaps even more valuable, when that person is most open to receiving help.
Just in time Adaptive Interventions identify when a person’s vulnerable 2 unhealthy influences and open 2 help.
Just-in-Time Mobile Interventions Should Leverage Behavior Change Research Evidence
The emergence of JITAI devices in the consumer universe and in the realm of eHealth poses important questions. How can we be sure these tools make good use of the evidence we have from behavioral research? How can we properly integrate our knowledge of health behavior and the mechanisms of behavior change into these tools? What processes are in place (or should be in place) to grow that knowledge with the data those tools are rendering available?
In a recent article for the special issue of the American Psychological Association’s Society for Health Psychology journal, Health Psychology: eHealth/mHealth, funded by the NIH Office of Behavioral and Social Sciences Research (OBSSR), Inbal Nahum-Shani and colleagues emphasize that these intervention assessments and delivery devices should be based and built on solid psychological constructs. They go on to suggest a framework for doing just that.
Can Just in time Adaptive Interventions #JITAI make good use of #behaviorchange research and evidence?
Citing existing and new evidence, Nahum-Shani et al., pose a workable model for key JITAI elements. JITAI fabricators must establish:
- The distal outcome (the ultimate goal of the tool);
- Proximal outcomes (the short-term goals that the intervention is intended to achieve, which are often mediators of the distal outcomes);
- Decision points (the array of possible options for type/dose/timing/delivery-mode of support selected based on currently available information); and
- Intervention options.
Tailoring variables include baseline and time-varying information that informs which intervention to offer at each decision point. Decision rules operationalize the individualization by specifying which intervention to offer at each level of the tailoring variable.
Identifying the Problem Before Building the Solution is Key for Just-in-Time Mobile Interventions
Nahum-Shani and colleagues are quick to point out that before developing and building the device, it is critical to formulate the problem under consideration: Who are you trying to help, what is the distal outcome, and what is the temporal progression of key factors toward the distal outcome?
B4 building Just in time mobile interventions, researchers must ID problem, desired outcome, how 2 get there.
Next, it is necessary to decide the adaptive strategy. This strategy should consider the factors that indicate the state of vulnerability, the appropriate interventions to use, and how these aids can be delivered precisely when the person is in the state of vulnerability.
The authors caution that given today’s data sets—which are literally made of hundreds of millions of data points—sophisticated data mining, machine learning, and other modern analytic methods will be needed so that JITAI developers can best capture user vulnerability and receptivity factors.
Read the Article
Building Health Behavior Models to Guide the Development of Just-in-Time Adaptive Interventions
Related Reads
Mobile Technologies to Manage Diabetes
About the Author
Wendy Anson, Ph.D.
Wendy Anson, Ph.D., is senior science writer and editor for the OBSSR at the NIH. She has written and developed literature reviews, book chapters, reports, grant sections, curricula, and award-winning educational films in the science and social science arena for medical schools, research hospitals, educational broadcasting organizations, and universities. Her Ph.D. is in educational psychology and technology.
Photo Credit: Shutterstock/ Denys Prykhodov